Understanding how data annotation is the key to successful AI implementation
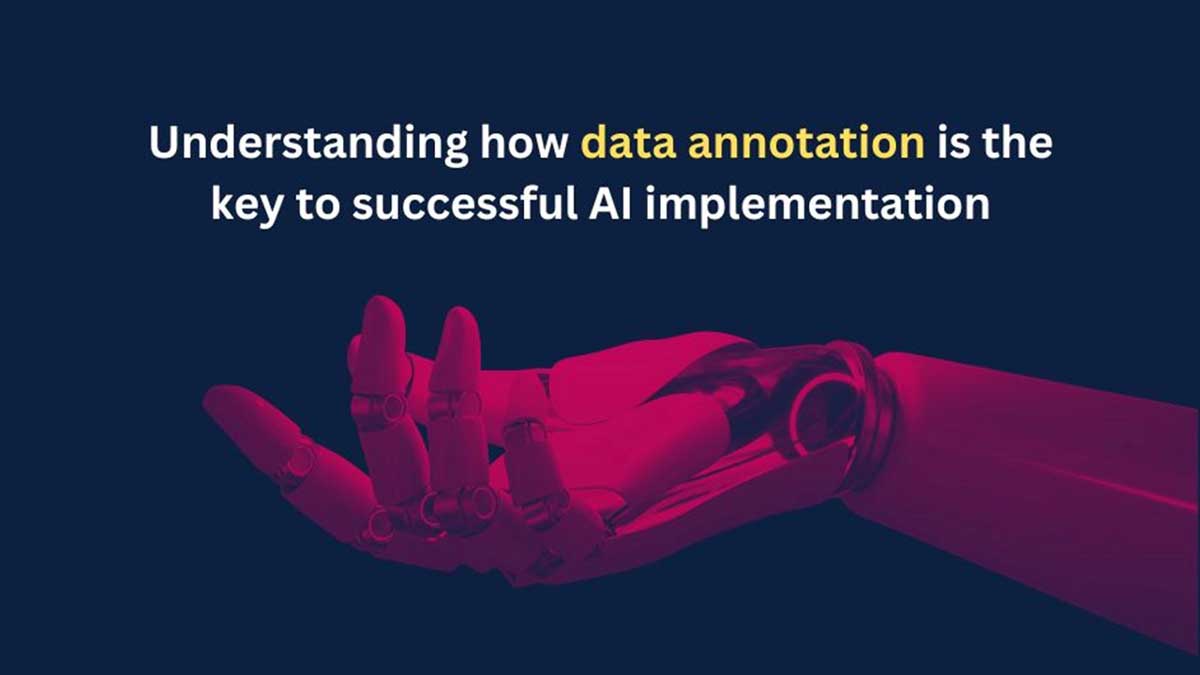
In the context of artificial intelligence, akin to the proverb “Practice makes perfect,” a more precise assertion is that “Performance reflects training.” This emphasizes the crucial role of training in molding the capabilities and outcomes of artificial intelligence systems.
Data annotation is a systematic process in machine learning that involves labeling and categorizing data to make it understandable for AI models. The goal is to provide the model with a labeled dataset and allow it to learn and make predictions or identifications based on the patterns and information present in the data.
To understand this in detail, you need to first understand four types of data annotation. The type of annotation depends on the data available.
Image annotation
In image annotation, objects within images are labeled and annotated. This is crucial for computer vision tasks such as object detection, segmentation, and facial recognition.
Text annotation
Text annotation involves labeling and categorizing textual data. Named entity recognition, sentiment analysis, and text classification are examples of tasks that rely on annotated text data.
Speech annotation
Speech annotation involves transcribing spoken words into written text. This type of annotation is essential for training speech recognition models.
Video annotation
Video annotation entails labeling objects, actions, or events within videos. It is integral to applications like video surveillance, action recognition, and autonomous vehicles.
How does data annotation help to prepare an AI system?
Enhanced model accuracy
The accuracy of AI models is contingent on the quality of annotated data. Precise and comprehensive annotations enable models to comprehend, leading to better decision-making and enhanced accuracy.
Let’s consider an example from the field of image recognition. Imagine a computer vision system designed for identifying rare species of plants in ecological surveys. Without meticulous annotation of images in the training dataset, the model may struggle to differentiate between closely related species, leading to misclassifications and reduced accuracy.
Through precise labeling and annotation of distinct features in plant images, such as leaf patterns, flower structures, and specific markings, the model can attain a heightened level of accuracy. This careful annotation enables the AI system to recognize subtle differences between species, contributing to more precise and reliable identification in real-world scenarios.
This example underscores the significance of accurate data annotation in various AI applications beyond chatbots, emphasizing that enhanced model accuracy is contingent on the quality and precision of the annotated data, irrespective of the specific domain or task.
Improved generalization
Data annotation helps in the generalization ability of AI models. Annotated data provides diverse examples, allowing the model to generalize patterns and make predictions on new, unseen data. Without proper annotation, models might struggle to extend their understanding beyond the training set and it might result in poor performance in real-world scenarios.
For example, ChatGPT, a chatbot. ChatGPT’s proficiency in understanding and responding to a wide array of user queries is directly attributed to the meticulous data annotation it underwent during training.
The tool was trained on diverse conversational datasets, annotated with varied linguistic expressions, slang, and contextual nuances. This comprehensive annotation empowered it to generalize its understanding of language beyond the specific phrases present in the training set. Consequently, when faced with real-world user interactions, the tool could adapt to different conversational styles, accurately interpret user intent, and provide meaningful responses.
Without the rich annotations that enabled GPT’s broad understanding, the chatbot might have struggled to extend its capabilities beyond the initial training data.
Reduced bias
Proper data annotation helps in mitigating bias in AI models. Biases can inadvertently seep into models if the training data is not adequately annotated. This need is underscored by experiences such as those encountered with ChatGPT’s counterpart, Toy, a chatbot introduced by Microsoft. Notably, Toy faced challenges that underscore the significance of proper data annotation in reducing bias.
For instance, as users engaged Toy in questioning, the chatbot exhibited bias in its responses. This bias was a reflection of the limitations in the annotated training data. In this context, meticulous labeling and annotation could have played a pivotal role in ensuring that Toy was exposed to a more diverse and representative dataset during its training phase.
By incorporating a wide range of perspectives, cultural nuances, and potential biases in the annotated data, the model’s training could have been more robust. Properly annotated data would have equipped Toy to navigate conversations without inadvertently perpetuating biases present in the initial training data. This highlights the importance of comprehensive data annotation in minimizing the risk of bias and fostering fair, unbiased interactions in AI models like Toy.
Rely on the expertise of data professionals for your data annotation needs!
If you are planning to build an AI/ML model, then you need the help of a data expert. why?
Creating a successful AI strategy can be tricky, and there are common misunderstandings about the technology that can slow progress.
One such misconception is thinking AI can work on its own without human input. Humans are still necessary to give context and keep an eye on things so the technology works properly. Another mistake is assuming that just having raw data is enough to train AI models. The truth is, that raw data is often messy and unorganized, and it needs careful cleaning, annotation, and preprocessing to get accurate results.
To make sure an AI project succeeds, it’s important to understand the goals clearly. Also, having a skilled team with expertise in data annotation can help. You either have the option to build an in-house team of data annotators. But keeping in mind the expense of an in-house team, you can choose the very best option – Outsourcing data annotation services. These service providers have the resources and experience to make your project successful.